Revolutionizing Defect Detection in Agriculture with AI and Machine Learning
Defect detection software, powered by artificial intelligence (AI) and machine learning (ML), is an exciting new technology that is changing the face of the potato processing industry.
The use of artificial intelligence in the food industry has been increasing over time, and one of the reasons it has become popular is due to the fact that some of the data it collects can be used to train machines to perform tasks.
In this article, we'll discuss how the agriculture/potato processing industry has evolved from manual detection to machine learning and AI; what exactly machine learning is; how it works on a technical level; how you can integrate AI into your warehouse's production line, and why it's a smart investment.
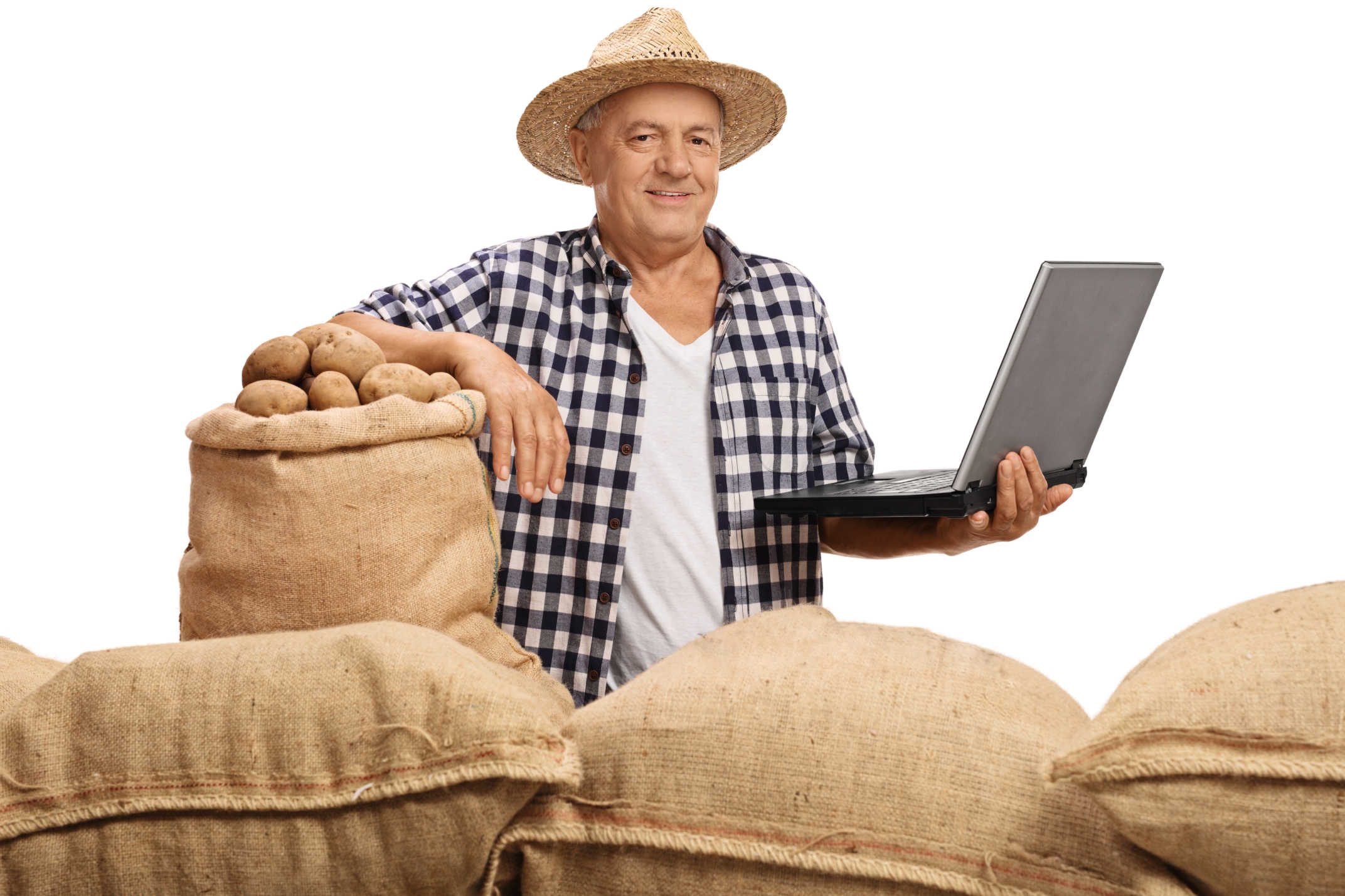
How the potato processing industry has evolved from manual detection to machine learning and AI.
The potato processing industry has evolved immensely over the past few decades. In the past, potato production was mostly manual and done by hand. Manual labor is effective and it is still used today, but is not always as efficient nor accurate when compared to automated machines.
The use of artificial intelligence in the food industry has been increasing over time, and one of the reasons it has become increasingly popular is due to the fact that some of the data it collects can be used to train machines to perform tasks. In this case, a machine learning model will be trained based on data from a specific category of produce, like potatoes. This allows the machine to "learn" how to pick out potatoes of a certain quality based on features (e.g. size and shape) that it has seen in previous images.
What is machine learning?
is a subfield of AI that allows computers to learn without being explicitly programmed. It’s most commonly used in image recognition, where it can distinguish between different objects and even different species, allowing the computer to identify images without being given specific instructions about what to look for. The computer, by itself, learns what it needs to look for and what constitutes an error, allowing it to adapt independently to new situations and data.
The idea behind this is pretty simple: When used by machines that are already present on production lines—for example, that view each item individually—AI can record and collect data about each individual potato or piece of produce that passes through. These machines can then send their data to the cloud so that an algorithm can begin bringing out patterns in the collected information. As the algorithm becomes more familiar with these patterns, it will begin to predict things like where bruising or decay may occur in individual items.
Features & benefits of agricultural processing machine learning
Features and Benefits of machine learning for agricultural processing include:
Large-scale crop defect detection
One way machine learning has changed defect detection in agriculture is through . Machine learning algorithms can be used to scan images of crops for signs of damage or disease. For example, an algorithm could be trained on images of to detect similar images when they appear in future crops. The same type of training can also be done for other kinds of defects—for example, identifying when the potato has been damaged or malformed.
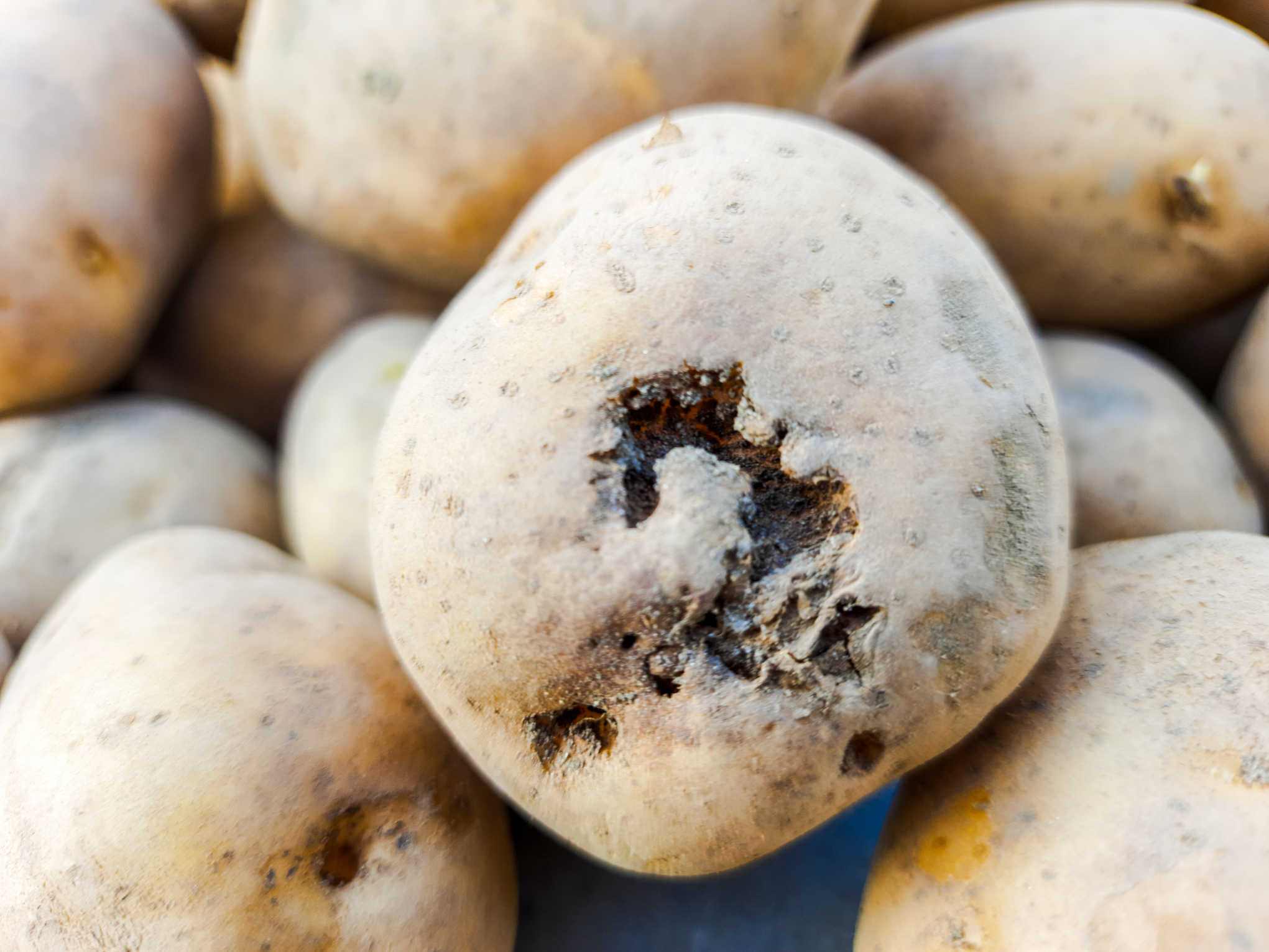
Yield and quality prediction through sensor data analysis
Sensor data analysis is another way machine learning changes agricultural defect detection. For example, sensors are used to about conditions on the farm—things like temperature, humidity, rainfall levels and soil moisture—and then those sensors send this information back to a computer where it's processed using machine learning algorithms which can then predict what kind of yield or quality might result from these conditions.
Automation
Machine learning can increase efficiency by automating processes like:
- Automating repetitive tasks that humans might find boring or difficult, such as collecting data from sensors in fields or processing data from weather stations.
- Decision-making abilities based on data and experience rather than relying on human intuition alone. For example, this can help farmers decide how much water or fertilizer to use on their crops.
- Using historical data to predict future outcomes and take proactive steps in response to those predictions (such as ordering more seed before it runs out).
Increased accuracy
Machine learning can be applied to any kind of data set in order to find patterns that would not be apparent otherwise. This gives farmers a more accurate picture of their operations and how they can improve them.
Eliminating Human Error
Machine learning algorithms don't make mistakes like humans do! This means they can analyze huge amounts of data much more quickly than humans can—and without making mistakes along the way (which would result in lower profits).
Cost-reduction
Machine learning can be used to optimize the growing process, which means that you will spend less time and money on things like watering and fertilizing, and more time and money on things that matter—like getting your crop to market.
Reducing waste and profit loss
The use of machine learning in agriculture has been shown to increase profits by up to 30%, due to the ability to identify optimal times for planting, harvesting, and processing. This can help farmers save money and reduce waste by harvesting only when it is profitable or selling produce during peak seasons when demand is highest.
Pepper Equipment's team of warehouse customization experts can help you integrate AI/ML software into your production line. .
Bantam Vision Grading System and Bantam Hollow Heart X-Ray: Best In Class Application of Artificial Intelligence/Machine Learning
The first step towards integrating AI/ML into your warehouse is choosing the machines and software that will work best for your company.
At Pepper Equipment, we will suggest the best software for your warehouse production line’s needs, size and output.
Bantam Vision provides a proprietary computer vision and machine learning system for grading and sizing potatoes. Additionally, it can be integrated with the Bantam Hollow Heart X-Ray.
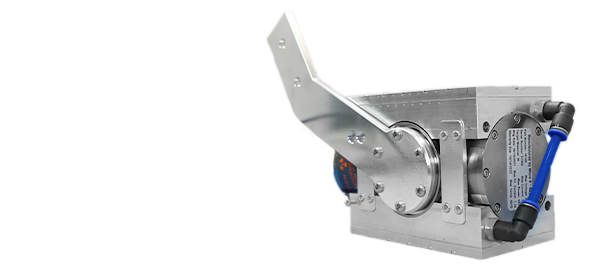
, an innovative grading solution that was first introduced in the potato industry in Monte Vista, CO in 2017, has been integrated with a potato warehouses’ current carrier system and offers significant cost savings as well as increased revenue for fresh-potato packing companies.
AI defect detection software is a smart investment for your potato processing warehouse production line
If you want to ensure that your potato processing warehouse is operating at peak performance, AI defect detection software can help. It can monitor the entire process, from harvest to packaging, and alert you if there is a problem anywhere along the way.
According to statistics reported by, The market for artificial intelligence in agriculture is growing rapidly, with spending expected to reach $4 billion by 2026. This is likely because global agricultural production . If the average farm generates an average of 500k data points a day, it only makes sense that these are the key market drivers for the integration of AI and machine learning into post-harvest agricultural production lines.
Pepper Equipment’s customers have seen tremendous benefits from using AI defect detection software. Are you ready to find out if this integration is right for your production line? .